Road to the future of driverless cars
-
- from Shaastra :: vol 01 issue 03 :: May - Jun 2022
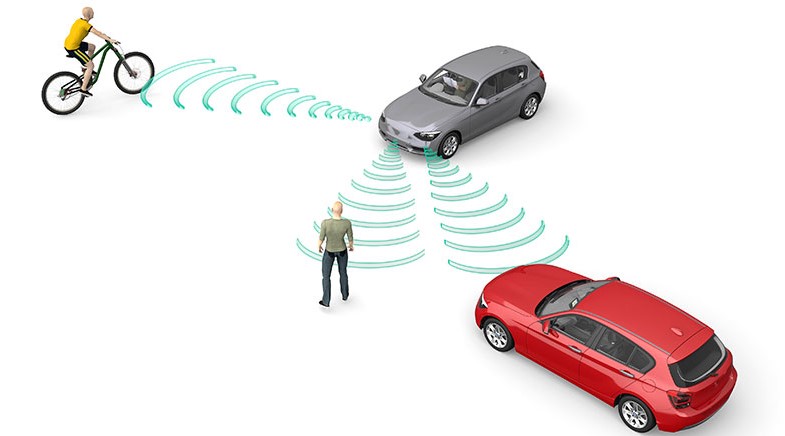
Recent research on enhancing safety surrounding autonomous vehicles.
IS IT A BICYCLE, A BIPED OR A BARRIER?
The era of driverless cars (or self-driving cars or 'autonomous vehicles') is nearer than we imagine, although they still have to overcome a few safety and regulatory hurdles before they get their engines revving. There is plenty of ongoing research around the world to marshal cutting-edge technology in the cause of enhanced safety surrounding AVs. Take object identification, for instance. AVs rely on sensors — cameras, radars and 'lidars' — for the decision-making that underlies autonomous navigation. And as Abhishek Balasubramaniam and Sudeep Pasricha at the Colorado State University note (in recent research supported by a National Science Foundation grant), one of the main tasks involved in "achieving robust environmental perception" in AVs is to detect objects in the vicinity of the vehicle using software-based object detection algorithms to determine if they are pedestrians, vehicles, traffic signs, or barriers. Other high-level tasks such as object tracking, event detection, motion control, and path planning hinge critically on this function. Object detection involves two sub-tasks: localisation, to determine the location of an object in an image; and classification, to assign a class ('pedestrian', 'vehicle', 'traffic light') to that object. Object detectors based on Deep Learning play a vital role in this. But these models are constrained by memory availability and processing capacity of on-board computers. The research rounds up the state of play with object detectors and the challenges for their integration into AVs. It also lays out how object detectors can be optimised for lower computational complexity and faster inference during real-time perception.
NAVIGATING IN FAIR WEATHER AND FOUL
The task of object detection (OD) and identification in AVs may be rendered more complex by "biases" in computer vision datasets owing to weather and lighting conditions, among other factors. Standard datasets used for training OD modules typically have only images captured in good weather conditions. When such data is used for OD module training, the model falters in respect of images containing weather 'corruptions'. Such biases have the potential to impair the dataset model's application, and render it ineffective for object detection in novel datasets. In real-life situations, this can have life-and-death consequences.

A recent study by researchers at the Pune-based Symbiosis Centre for Applied Artificial Intelligence, and the Chongqing University of Posts and Telecommunications, China, focuses on understanding these datasets better by identifying such "good weather" bias, and demonstrates methods to mitigate such biases, thereby improving the robustness of the dataset models. The study proposes an effective OD framework to study bias mitigation, and uses the framework to analyse the performance on popular datasets. To mitigate the bias, it proposes a knowledge transfer technique and a synthetic image corruption technique. The findings were validated using the Detection in Adverse Weather Nature (DAWN) dataset, which is made up of real-world images collected under various adverse weather conditions. The study claims that the experiments show that these techniques outperform baseline methods several-fold.
WATCH OUT FOR EYE CONTACT IN THE WILD!
On the road, whether you're walking or driving, eye contact with others is critical for safe navigation. It signals situational awareness and communicates intentions. A person staring into a handphone is less likely to be aware of their proximate environment, and for that reason may prove a hazard - to themselves and to others. Autonomous agents - such as driverless cars and robots - too have an 'eye' of sorts, and they need to understand the implicit channel of communication through eye contact in order to avoid collisions and move smoothly around humans. For instance, a driverless car must figure out whether a pedestrian intends to cross the street in front of the vehicle or yield way. Likewise, robots moving among crowds of people should be able to detect whether pedestrians have noticed them - or will likely collide with them. Detecting eye contact 'in the wild' (that is, in situations with no constraints on or prior knowledge of the environment) presents challenges, since the contact can be quick and subtle, with small head movements lasting barely a few milliseconds. Although eye contact in the wild is a critical part of safe navigation of AVs, its study has not received quite the same attention as other aspects such as object detection.

A recent paper, titled 'Do Pedestrians Pay Attention? Eye Contact Detection in the Wild', by researchers at Sorbonne University, France, and at the Visual Intelligence for Transportation (VITA) lab at EPFL university in Switzerland, addresses this area. Since existing datasets "are not sufficiently diverse" for data-driven methods, the researchers created a new large-scale dataset, called LOOK, for eye contact detection in the wild. (The source code and the dataset are publicly shared.) They also propose a Deep Learning model leveraging semantic keypoints, specially adapted to eye contact detection; additionally, they suggest an evaluation protocol for eye contact with real-world generalisation. The study claims that this approach yields "state-of-the-art results" and strong generalisation. "We hope that this new benchmark can help foster further research from the community on this important but overlooked topic," they conclude.
THE CAR THAT READS PEDESTRIANS' MINDS!
Driving on busy streets, alongside pedestrians and vehicles of various kinds, requires not just technical prowess, but also a social skill of sorts. In some ways, you have to be a mind-reader, second-guessing other drivers and cyclists - as they weave in and out of traffic or whimsically change lanes - and occasionally distracted pedestrians. AVs too have to cultivate a similar 'mind-reading' capability in order to judge the goals and intents of different road users, not just as a one-time function, but continually. It's fair to say that eternal vigilance is the price of accelerated mobility. There are several algorithms to predict, say, pedestrians' behaviour - especially trajectory prediction and action recognition. These algorithms make driving decisions based on predictions of pedestrians' trajectories and optimise decisions to avoid crashes. Vehicle dynamics are usually calculated based on instantaneous motion parameters of all the involved objects or learning-based models to predict the future trajectories.

However, as a recent study by researchers at Indiana University - Purdue University Indianapolis (IUPUI), and Tulane University, observes, several "fundamental limitations" still obstruct smooth and efficient interactions between AVs and pedestrians. The study, titled 'PSI: A Pedestrian Behavior Dataset for Socially Intelligent Autonomous Car', and sponsored by the Collaborative Safety Research Center at Toyota Motor North America, seeks to fill this knowledge gap by creating a benchmark Pedestrian Situated Intent (PSI) dataset. As part of video experiments for cognitive annotation, human drivers with diverse backgrounds were recruited. The dataset, which is in the public domain, generated labels for the dynamic intent changes for pedestrians crossing in front of the subject vehicles, and the drivers' explanation process to account for their estimates of pedestrian intentions and predictions of their behaviour. The study claims that these cognitive labels "can fundamentally improve the current designs of pedestrian behavior prediction and driving decision-making algorithms towards socially intelligent AVs."
Have a
story idea?
Tell us.
Do you have a recent research paper or an idea for a science/technology-themed article that you'd like to tell us about?
GET IN TOUCH