Fighting an infodemic
-
- from Shaastra :: vol 01 edition 02 :: Jul - Aug 2021
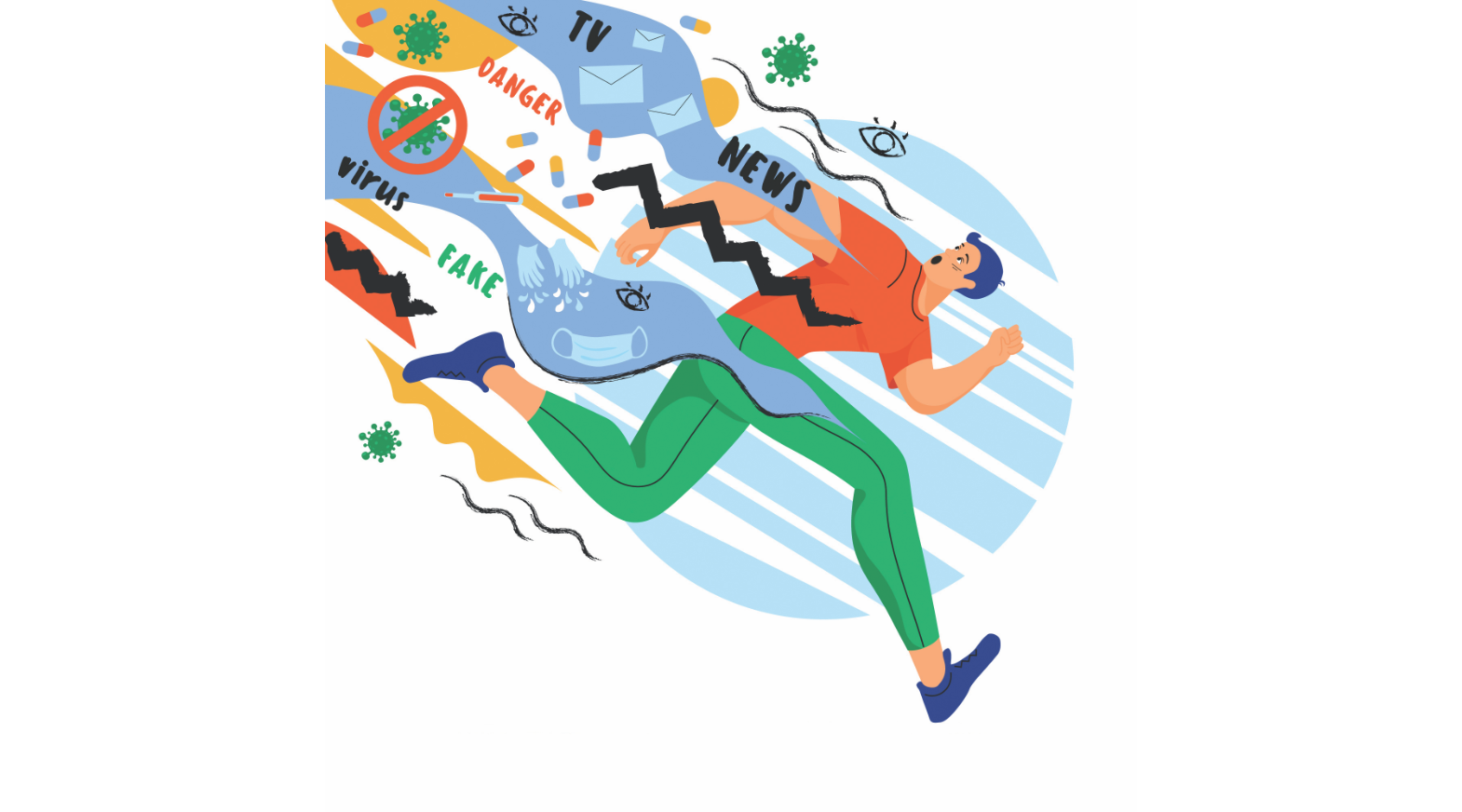
The disinformation epidemic is as virulent as COVID, and just as lethal. But technology can help keep public platforms safe.
In August 2020, when COVID-19 was raging across the world, World Health Organization Director-General Tedros Adhanom Ghebreyesus raised an additional red flag. "We're not just battling the virus," he said. "We're also battling the trolls and conspiracy theorists that push misinformation and undermine the outbreak response." An 'infodemic' — an information epidemic — was feeding into anti-vaccine sentiments and pushing people to take unsafe steps in the hopes of a cure, the agency noted. In Iran, nearly 700 people died after drinking toxic methanol based on social media claims that it could kill the virus. "This is the kind of dangerous misinformation that WHO is most worried about," the organisation said.
Infodemics spread even in 'normal' times. In 2017, there were several instances of lynchings in Jharkhand: vigilante mobs, triggered by WhatsApp rumours of children being kidnapped, had set upon suspected 'child-lifters'. Over 20 people were killed, and over 600 arrested.
Some 11,000 km away from India's heartland, in Pennsylvania, S. Shyam Sundar read reports of these lynchings in horror. As a doctoral student at Stanford in the 2000s, he had studied the impact of the internet on media and society, but for the first time in years, he was watching misinformation risk lives. "It was mostly an academic pursuit in the 1990s, but now fake news and misinformation have devastating real-world impact," he told this writer.
By most accounts, the battle against the infodemic is proving arduous. But technologists and organisations are coming together to combat the infodemic, and early results are promising. "The only way to deal with this infodemic at scale is to build guardrails with technology," says Sundar, founding director of the Media Effects Research Laboratory at Penn State University's College of Communications.
The roots of the 'fake news' problem run deep. Even before the internet era, misinformation and fake news were used to sabotage political opponents. In the 1970s, investigative journalists Bob Woodward and Carl Bernstein made public the political 'dirty tricks', including active disinformation, at play in the Watergate scandal. News (including fake news) did not travel as fast in those times; even when the internet became mainstream, fake news spread mostly on email. Websites that countered misinformation were good enough for the time. Email providers also got better at fighting spam. But with cheap data, smartphones and social media in the hands of billions of users, the speed and scale at which fake news travels and snowballs into a bigger problem have accelerated.
The year 2016 was a tipping point. A BuzzFeed News analysis noted that leading up to the U.S. Presidential elections that year, fake election news had more engagement on Facebook than election stories from 19 top news outlets taken together. "This made people sit up and take note of the fake-news phenomenon," says Sundar. Several hyper-partisan pages on Facebook and more than 100 U.S. politics websites that operated out of Macedonia, in the Balkans, published false or misleading content at an "alarming rate". Underemployed Macedonian teenagers were making a quick buck by driving traffic to their fake-news sites.
Subsequent investigations established that fake news was being used by several agencies, including foreign ones, to undermine democracy. "This became an international phenomenon. It's a big issue in India as well, and extends beyond politics," says Sundar. The internet has made it possible for anonymous users in one part of the world to influence millions of others elsewhere.
TECHNOLOGY & SOLUTIONS
When companies like Facebook, Twitter and Google were held responsible for amplifying the infodemic, they took several remedial measures. The most important one was to create a network of third-party fact-checkers and content moderators who could verify stories and help flag them. Several such initiatives have mushroomed across the world. Some are independent; some are funded by platforms. The International Fact-Checking Network (IFCN) was set up by the Poynter Institute in September 2015 to support fact-checking initiatives by promoting best practices. India has more than a dozen fact-checking outfits, including BOOM, WebQoof, THIP Media and Alt News.
"At present, technology can only be assistive and not proactive," says Rajneil Kamath, co-founder of Newschecker, an independent fact-check initiative that’s part of the IFCN. "Many of our processes and tasks are being automated," says Kamath, whose company fact-checks news in nine Indian languages.
However, not only is human fact-checking and content moderation hard to scale, it also takes a toll on people. Workers hired to moderate content on platforms develop psychological problems from prolonged exposure to harmful content. Between third-party fact-checkers and outsourced content moderators, only a fraction of the content online is checked. "By the time it’s fact-checked, the damage is already done," says Sundar.
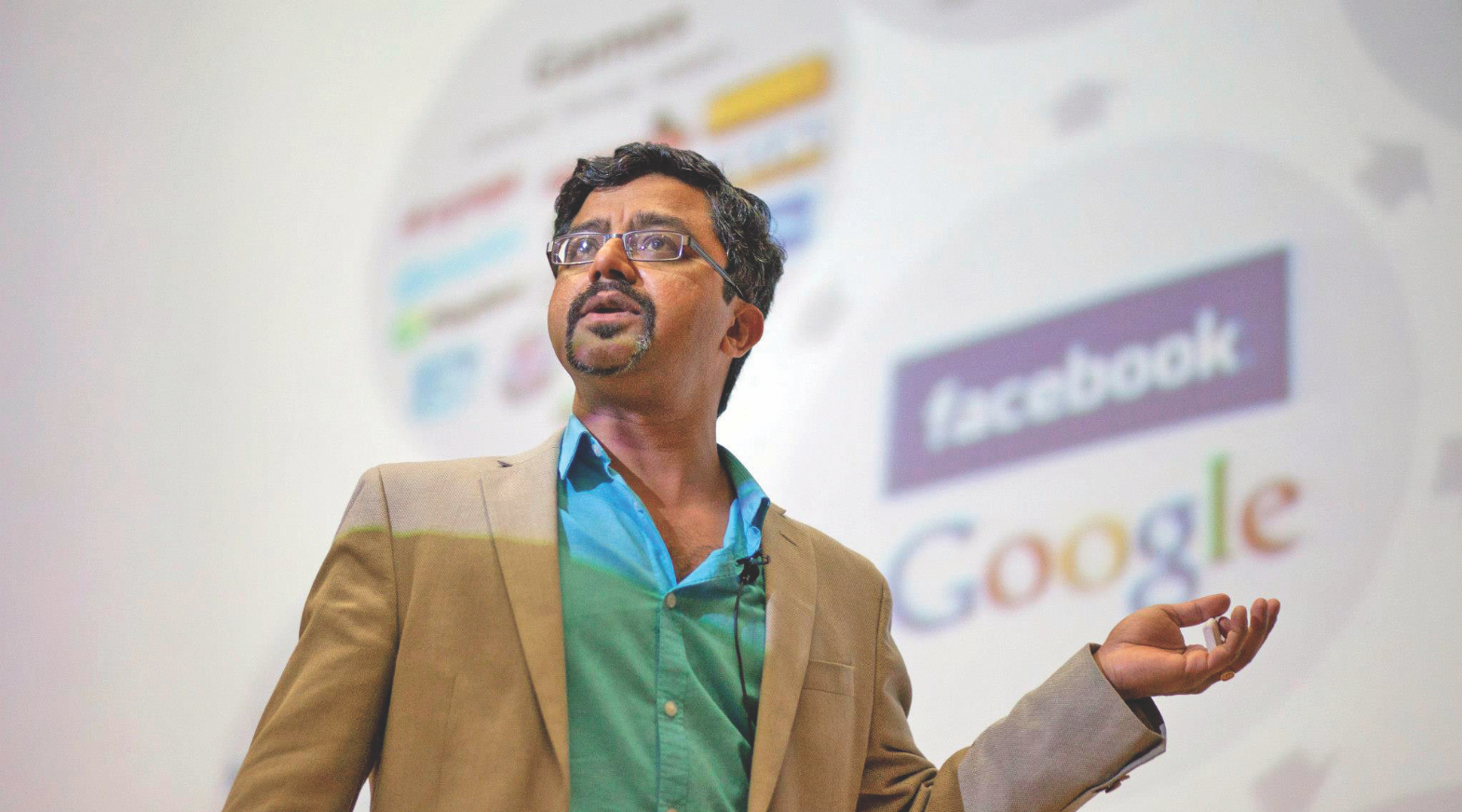
“The only way to deal with infodemics at scale is to build guardrails with technology," reckons S. Shyam Sundar.
To slow the velocity of misinformation and fake news, platforms have also built product features. WhatsApp, for instance, introduced a limit on forwarding viral messages. Twitter prompts its users to read an article before it's reshared on the platform. This has had some impact: Twitter learnt that people access articles 40% more often after seeing the prompt. But the volume of fake and misleading information is still high.
This meant that platforms lean more and more towards technology that helps look for and flag tell-tale signs of fake or misleading information. The signs could be the way sentences are structured, the source of information, and dozens of other factors. Even so, fake stories spread, and organisations have to take proactive measures to curb misinformation. WHO's response is a good example of such an approach. It worked with nearly 50 tech companies, including TikTok, Google, WhatsApp and YouTube to prompt warnings and prioritise information from official sources to users. Over 1 billion people were steered towards COVID-related resources from health authorities after seeing Facebook prompts, the company said in March.
WHO also partnered with an analytics company, which reviews nearly 1.6 million pieces of information on various platforms and uses machine learning to glean insights into what users are searching for and comes up with tailored messages. It also collaborated with the U.N. Global Pulse Initiative to listen to radio news in some countries, and used speech recognition to identify and address concerns.
At the other end, researchers like Sundar are beginning to understand the problem better. His proposal to examine the Jharkhand lynchings was funded by WhatsApp, which set aside $1 million to fund research across five areas, including information processing of problematic content; digital literacy and misinformation; election-related misinformation; network effects and virality. The project, titled ‘Seeing is believing', found that videos were considered more believable. In 2019, Sundar's team tested the hypothesis by stripping down the story into audio-only, and into text-only. The messages were shown on WhatsApp to 180 participants in Delhi and Bihar split across urban and rural areas. The study found that people who don't know much about a topic are more likely to fall for the "video effect".
"Video fake stories are more pernicious because they seem to make people believe they have seen it," says Sundar. The team recommended that platforms prioritise action against video fakes. This, of course, throws up newer challenges because synthetic videos are also growing at an exponential rate. The growth is driven in part by the use of artificial intelligence techniques to generate so-called ‘deep fake' videos – "fake" videos created using "deep" learning.
"It is a recipe to generate fake news at scale. And scale makes it harder to deal with," says Dr Sundeep Teki, who has worked on artificial intelligence problems at Amazon Alexa and Swiggy.
While public platforms like Twitter and Facebook are able to see the content and take action on it, platforms like WhatsApp are constrained by their encryption commitment and the expectation of privacy of communication. One way to intervene is to base their actions on meta tags, which can tell videos from text. Flagged videos can be marked for more active investigation and study.
AI vs FAKE NEWS
The most promising way to deal with the infodemic at scale is the use of artificial intelligence and machine learning. The idea is to label stories and users as fake or real or flag them for human investigation using machine learning models. Researchers concur that differentiating between a real and a fake story is a fairly contained problem. But once a story is identified as fake, the job gets harder. 'Stories' can be political commentary, satire, opinions, native advertisements and so on. It is hard to differentiate between satire and patently false information with intent to harm, and between native advertising and opinion pieces.
Techniques such as supervised learning algorithms that are built for English can be scaled to other languages (even if some nuances are missed) by taking a corpus of material and training the models using new data. For example, a big part of an ongoing project at Facebook identifies the language in which the content is created. The project, funded by a Social Media and Democracy Research Grant, awarded by The Social Science Research Council, will let researchers see the websites that users have shared, unique characteristics of the URLs, and some demographic information about users.
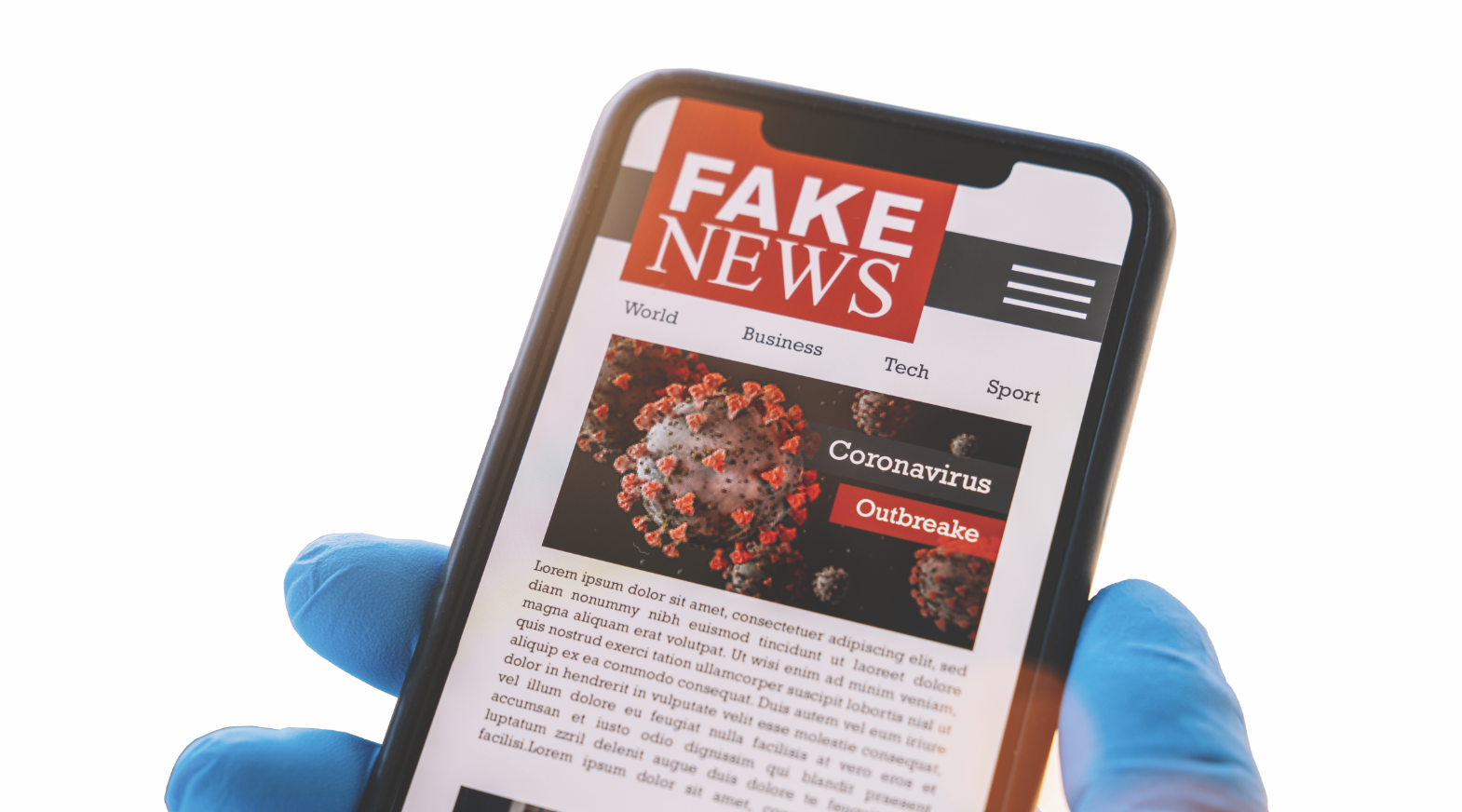
THE DATASET CHALLENGE
While fake-news models can be applied to nearly every language, platforms may not drive these initiatives due to market considerations. This is where the work of experts like Teki becomes important. Teki has worked in four countries at the intersection of artificial intelligence and neuroscience. He was also a Wellcome Trust Fellow in Neuroscience at Oxford University and obtained his PhD from University College London. Along with student collaborators, he recently published two papers focussed on COVID-19 fake-news detection and hostility detection in Devanagari (Hindi) Tweets at the 'NLP for Internet Freedom Workshop', COLING'2020, and 'Combating Online Hostile Posts in Regional Languages during Emergency Situation', AAAI 2021.
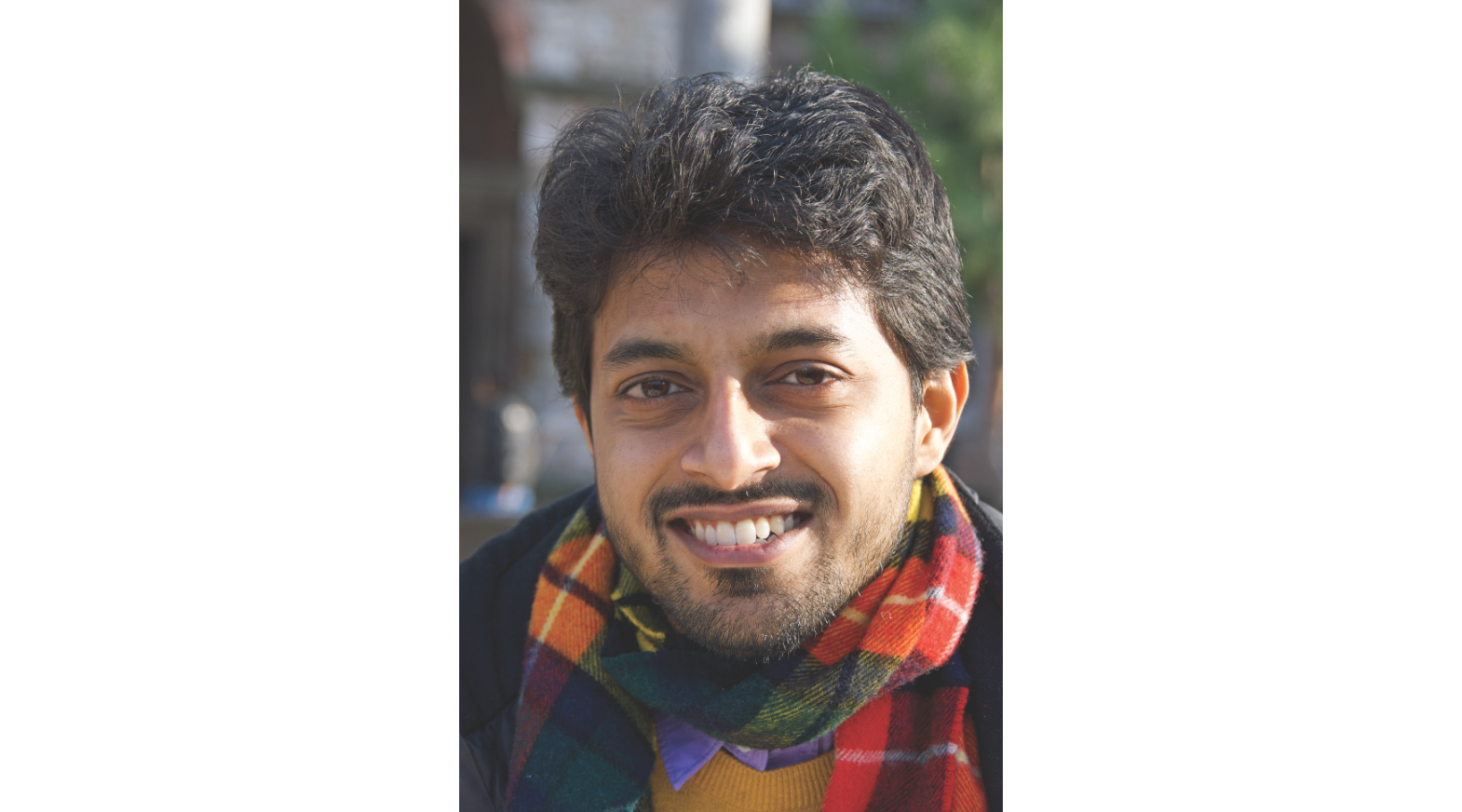
"Sophisticated deep learning models can be used to detect fake news and fact-check for emerging topics like COVID-19," Teki says.
Artificial intelligence models use large amounts of publicly available text (like Wikipedia) to train established 'language' models such as GPT-3, BERT or ALBERT. As these models process more data specific to a problem, they learn to better understand the relationship between words and the context of their use. For instance, Bidirectional Encoder Representations from Transformers (BERT) is a natural language processing (NLP) model developed by Google in 2018. It was mainly used to understand user searches and was a landmark breakthrough in NLP. Compared to previous language models, BERT demonstrated state-of-the-art performance on a variety of natural language understanding tasks by capturing more sophisticated contextual relationships between words. If you say: "There's a coronavirus lockdown in Bengaluru," the model understands that Bengaluru is a city, coronavirus is a pandemic, and so on. These models serve as a proxy for a language, to better capture the meaning and the context. "The holy grail for work on language models is to improve their contextual understanding of words and sentences to the same level as humans, and eventually surpass human benchmarks," says Teki.
"Deep learning models can be used to detect fake news and fact-check for emerging topics like COVID-19," says Dr Sundeep Teki.
Once the researchers zeroed in on a language model, they applied a technique called transfer learning. The idea was to parse through thousands of new sentences particular to COVID-19 and get a better understanding of those sentences. "Once we have a piece of text in a vectorial form (embedding) it is easy to do all kinds of mathematical, machine learning approaches to understand and predict various aspects of language, including intent, sentiment, entities, comprehension, and so on," Teki explains. More than nine times out of 10, the model could identify whether a Tweet was fake or not. The models get better with more powerful algorithms and better-quality data. Several fake-news detection datasets are available on Github, but these are mostly focussed on the U.S. and Europe. Manually curating such datasets is difficult and prone to errors: ImageNet, the most popular image recognition dataset, was built over 4-5 years. "We don’t have robust data sets like ImageNet for fake news yet," says Teki.
OPEN DATA AND RESEARCH
With platforms opening up data for researchers, things might improve in the future. For instance, over 100 researchers and developer teams were granted access to the COVID-19 data stream by Twitter. More than half of them focussed on disinformation and misinformation around the coronavirus. Open data from platforms can also throw up interesting findings that help combat the infodemic. By using data from Twitter and artificial intelligence techniques, researchers from Georgia Institute of Technology and New York University created a novel dataset of 155,468 COVID-19-related tweets, containing 33,237 false claims and 33,413 refuting arguments. Their findings, published in November 2020, yielded an important insight: to effectively study misinformation, one needs to tap into the wisdom of the crowds "because 96% of all refutations are being done by concerned citizens (i.e., the crowd)." The "crowd" often counters misinformation with links to fact-checks or other trusted sources. Opinion-based tweets were more assertive, used more negative words, were more abusive, and exhibited negative emotions and anger, the study found. The analysis can potentially lead to the development of better tools and an understanding of approaches that use the crowd to counter misinformation.
While these are promising approaches, technology alone can't solve the infodemic in its entirety. It will need a multidisciplinary approach with researchers, policymakers, platforms and technologists coming together.
Have a
story idea?
Tell us.
Do you have a recent research paper or an idea for a science/technology-themed article that you'd like to tell us about?
GET IN TOUCH