Tech tools that help map landslides
-
- from Shaastra :: vol 03 issue 10 :: Nov 2024
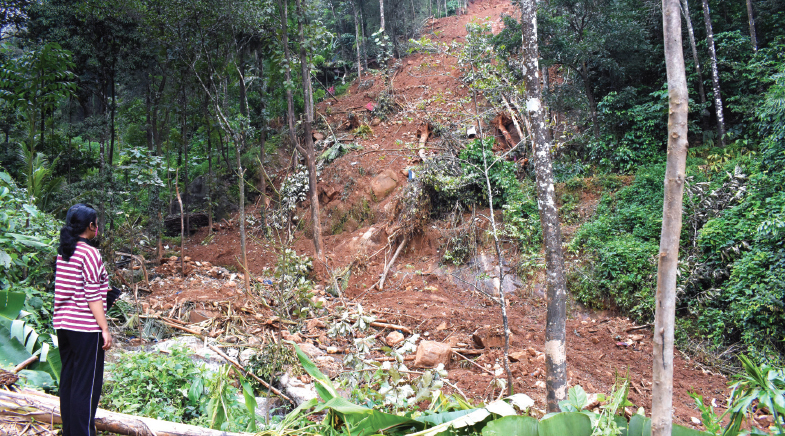
Researchers are using technological tools, including AI/ML, to map landslide risks and to sound early alerts.
Growing up near Meenachil River in Kottayam district of Kerala, Minu Treesa Abraham had had up-close experiences of the perils of floods induced by landslides. That lived experience was one of the reasons for her securing a Junior Research Fellowship in the Geohazards Research Lab at the Indian Institute of Technology Indore in 2019 under Prof Neelima Satyam. "She knew I would put my soul into it," reckons Abraham, now a Project Engineer at the Norwegian Geotechnical Institute (NGI).
In late July 2024, when a series of landslides wracked Wayanad district in Kerala, killing over 300 people and causing immense devastation, her professional interest was piqued. "We knew that landslides would happen in these areas, but not at this level," recalls Abraham. Based on landslide susceptibility maps prepared by the National Centre for Earth Science Studies, Thiruvananthapuram, and other researchers, scientists had long warned of such a tragedy.
Back in 2019, Abraham had been looking to use rainfall data to predict landslides in her home State. Landslides were a recurring feature of Idukki district, and Abraham was keen to establish what level of rainfall had triggered landslides in the past – and use that to predict future landslides. "I collected data from the Geological Survey of India and State government reports from 2010 to 2018 to identify where and when landslides can occur based on the spatial conditioning factors and a triggering mechanism like rainfall," says Abraham.
Establishing a correlation between historical data on high-intensity rainfall and the occurrence of landslides is useful. If the data determines that a threshold value of rain intensity triggers a landslide, civic systems can be designed to set off an alert of a possible landslide whenever rainfall intensity exceeds that threshold.
However, in 2019, Idukki had only four rain gauges. A wider network of gauges was needed for accurate prediction of landslides.
A year before Abraham started collating historical rainfall data, geologist Sajin Kumar K.S. was at a conference in the U.S. to convince fellow geologists of the need to install more rain gauges at sites at high risk of landslides in Idukki district. Persuaded by his pitch, the Society of Exploration Geophysicists (SEG), a global non-profit organisation, committed itself to funding 25 rain gauges.
"I have installed rain gauges at eight locations in Idukki, and look for rainfall data from IMD weather stations," says Kumar, now an Assistant Professor of Geology at the University of Kerala. Data can be generated with a latency of 5-30 minutes, he adds. With the university's support, he has designed an app that flags landslide vulnerability based on rainfall data collected by the SEG-funded rain gauges.
Systems can be designed to set off an alert of a possible landslide whenever rainfall intensity exceeds a threshold.
That app, called Slope Instability Predictor–Kerala (SLIP-K) uses Google Earth Engine (which harnesses satellite imagery and geospatial datasets) to send out push notifications when rainfall exceeds a threshold value. That value is a sliding scale, calculated by reviewing the rainfall data that triggered landslides in the past. For instance, the app starts with a green interface. It turns yellow when the rainfall exceeds 25% of the threshold value; orange at 50%; and red at 75%. In July 2024, SLIP-K correctly predicted landslips on the Munnar Gap Road.
OTHER INPUTS NEEDED
Having adequate rain gauges is critical, but to predict landslides, a cache of other information, too, is needed. "Information on soil and hydrological conditions is (also) crucial," says Roxy Mathew Koll at the Indian Institute of Tropical Meteorology, Pune.
Researchers in Norway have been digging deep for just that kind of information. In a 2022 study (bit.ly/landslide-soil), Luca Piciullo and colleagues at the NGI used sensors placed at various depths in landslide-prone areas to understand the soil's nature as it soaks up rainwater.
According to Piciullo, these sensors gather hydrological data such as pore water pressure and volumetric water content along with measurements of atmospheric pressure, precipitation, solar radiation, air temperature, and vapour pressure. This data trove, monitored over a year, was used to model a machine learning (ML) algorithm for a slope in eastern Norway.
To put all the data in one place was challenging. The team developed an NGI live dashboard with data from meteorological stations. Using the data, the team back-calculated to match the results to test the robustness of the model using the Random Forest algorithm, a powerful ML tool for regression analysis. The study emphasises the importance of constant monitoring of soil conditions.
Abraham points out that typically, sensors are specific to a Local Landslide Early Warning System (Lo-LEWS), and to expand the warning system to a broader region, researchers look for other methods – such as a large landslide susceptibility map.
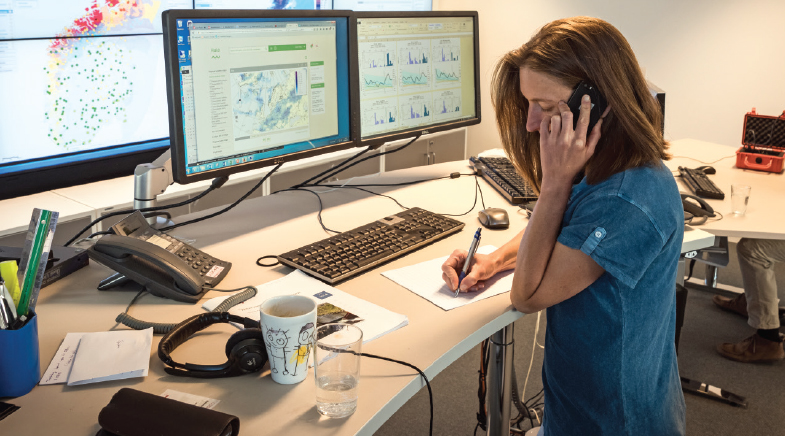
In January 2024, Manabendra Saharia and Nirdesh Sharma in the Department of Civil Engineering at the Indian Institute of Technology Delhi drew up a landslide map of India with details of soil patterns and human settlements (bit.ly/landslide-iitd). It uses an ML algorithm to flag the landslide susceptibility of places even without a history of landslide, up to a resolution of 100 metres. They have since published details of their efforts to build ML-CASCADE, an open-source tool designed for real-time landslide mapping (bit.ly/map-cascade). The authors claim this tool will obviate time-consuming, labour-intensive field studies and manual mapping.
THE HUMAN ELEMENT
According to Graziella Devoli, Senior Geologist at the Norwegian Water Resources and Energy Directorate, an efficient early warning system is adept at releasing warnings only with an organised structure with people and the timely updated threshold value of landslide-triggering factors.
Devoli is part of a team that in 2013 launched a Norwegian Forecasting and Warning Service for Rainfall and Snowmelt-Induced Landslides. On Varsom.no, a Norwegian web portal, details of landslides on a regional scale are published daily. The warning system involves not only weather alerts and data but also a human, who decides whether or not to send a warning and what level of risk to flag.
The system works based on five pillars: "automated hydrological and meteorological stations, landslide and flood historical database, hydro-meteorological forecasting models, and a trained group of forecasters," according to a 2018 paper (bit.ly/norway-forecast).
Devoli acknowledges the difficulty in registering every landslide, and reckons this reinforces the need for more systematic mapping effort after rainfall events.
Erin Lindsay, a PhD student co-supervised by Devoli, therefore harnesses artificial intelligence (AI) to map landslide events using satellite data. In her thesis, Lindsay claims that use of satellite data overcomes "spatial bias" – where only landslides in populated areas are recorded. Lindsay produced an inventory of about 120 landslides triggered by extreme rainfall in western Norway in July 2019.
IIT Delhi researchers have drawn up a landslide map of India, and built a machine learning-based tool for real-time risk mapping.
The use of ML models has advanced progress in landslide prediction. A June 2024 study (bit.ly/ML-landslide) considered the dynamic nature of events into the model, to update the map on present conditions such as rainfall. "This approach enables changes in landslide probability as new rainfall events occur," says lead author Nicola Nocentini, a Research Fellow in the Department of Geosciences, University of Padua, Italy.
Nocentini says, "The model continuously updates based on the relationship between rainfall and historical landslide occurrences to provide real-time predictions of landslide probability. So, the model refines its predictions by learning from the entire dataset, including new landslide incidents when they become available." Drawing up a landslide inventory is crucial for this ML approach, as it provides the historical basis for training the models and enhances the accuracy of predictions.
However, there is also a compelling need for well-documented databases. For instance, information on the exact date of occurrence of a landslide is critical, as it allows researchers to correlate an event with the triggering rainfall.
In Nocentini's estimation, the most challenging step in this field is understanding and adding information on the intensity of landslides (mass, volume, and velocity) to estimate the impact of a disaster.
However, others believe landslide prediction technology can only take you so far. According to Sekhar Kuriakose, member-secretary of the Kerala State Disaster Management Authority, all this reinforces the need for continuing research in early warning systems and technology. AI models have to be "handy to use" specifically for a particular area, he reckons. "As a disaster manager, I will not invest disproportionately in landslide early warning systems and trust them to give minute-by-minute warnings," he says.
In his assessment, in order to prevent landslides, "we need to strengthen local governments and create awareness among the public regarding understanding threatening disasters. Then, as an icing on the cake, we can build an early warning tool with tools like sensors."
See also:
Have a
story idea?
Tell us.
Do you have a recent research paper or an idea for a science/technology-themed article that you'd like to tell us about?
GET IN TOUCH