The transformative power of AI
-
- from Shaastra :: vol 03 issue 01 :: Jan - Feb 2024
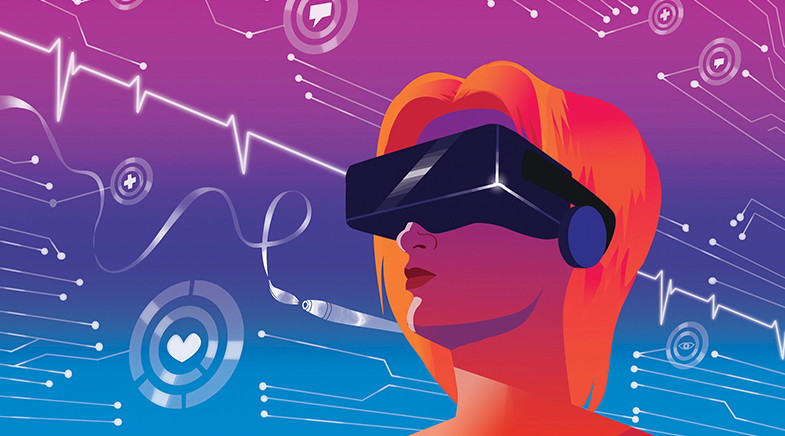
Artificial intelligence systems are transforming industrial and sectoral landscapes. How soon before they outperform humans?
In the middle of the 20th century, mathematician Alan Turing introduced a concept that continues to define computer intelligence. If a computer fools you into thinking it is human, it shows intelligence. In the 70-plus years since, Artificial Intelligence (AI) has transformed from learning chess to defeating grandmasters at their own game; from recognising unlabelled images of trees to painting them on cue.
One of the attributes of a human being, perceived as a marker of intelligence, is the ability to make decisions. AI researchers thought that if certain explicit rules and conditions were taught to a computer, it could also make decisions. In 1955, Herbert Simon and Allen Newell introduced Logic Theorist, a computer program designed to replicate the reasoning used to prove mathematical theorems. Logic Theorist is considered to be among the first AI programs.
About the same time, computer scientist John McCarthy first used the term 'Artificial Intelligence'. Soon, programming languages, using list processing to manipulate data, were developed to write AI programs. This eventually led to the creation of an early predecessor of chatbots called ELIZA by MIT's Joseph Weizenbaum in the mid-1960s. ELIZA could participate in conversations by replicating speech patterns, but with no real understanding of what was being said.
The growth of AI runs parallel to the growth in the generation and processing of huge datasets. With the democratisation of the Internet in the last three decades, unstructured data began accumulating – from online transactions to social media posts and wearable sensor information. Today, over 2.5 quintillion bytes of data are being generated every day. To store all this data and make sense of it, techniques like cloud computing were developed. By the 2010s, a synergy blossomed between data science and AI. Big data analytics laid a foundation for training AI models, and conversely, AI made it easier to collect and process data.
In the 2010s, deep learning involving multilayered neural networks was used to train computers to follow decision-making patterns similar to those of humans. Where traditional machine learning with a single neural network relied on labelled data, the presence of three or four neural networks could process unstructured data and extract patterns. This was facilitated by zero-shot learning, a technique that helps models classify previously unseen data without training. This improved algorithms for object detection and image classification. Unlabelled images of cats were among the first to be recognised by a Google-developed neural network in 2012.
The ImageNet project, a visual database started by Stanford data scientist Fei-Fei Li in 2007, has played a key role in advancing computer vision. A visual database for researchers to benchmark their machine learning (ML) models, it has collected over 14 million annotated images so far. Meanwhile, research in speech recognition and generation brought forth the era of voice assistants like Siri and Alexa.
PAST ISSUES - Free to Read
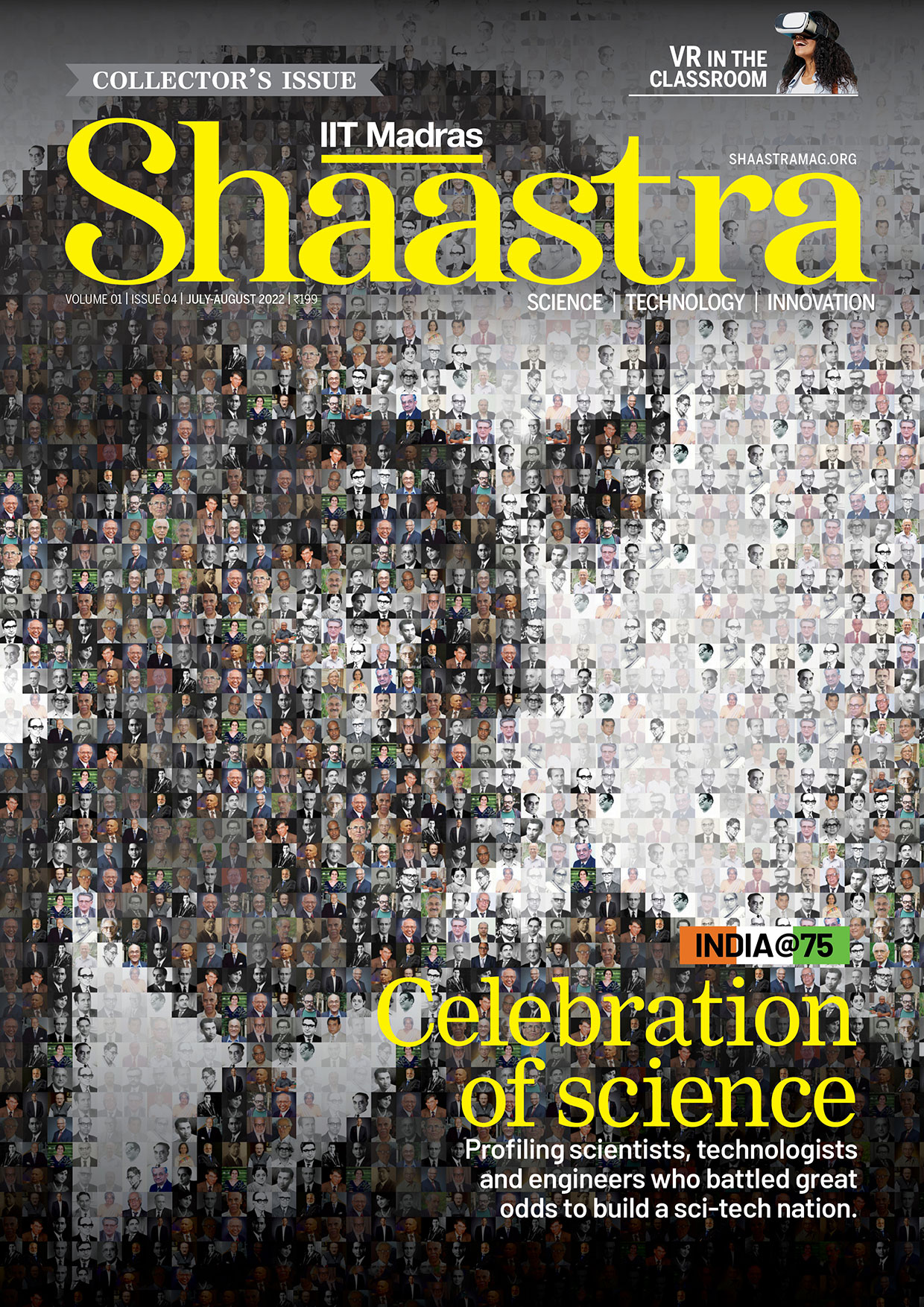
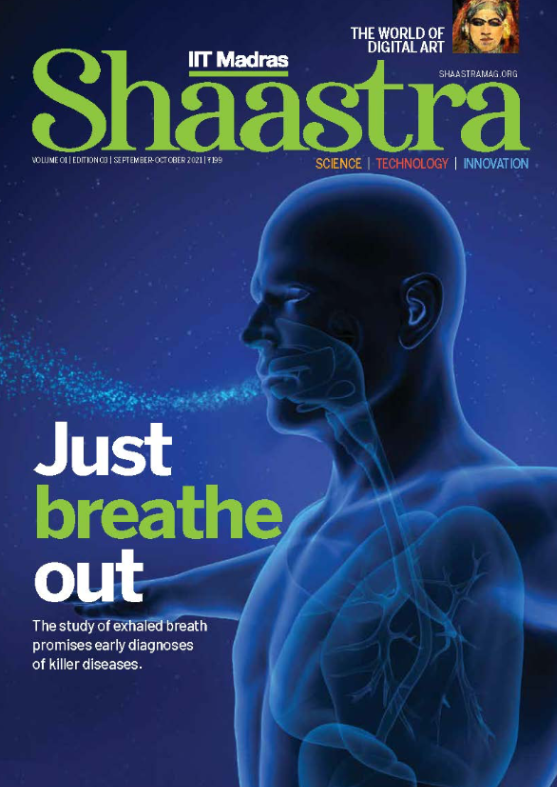
Have a
story idea?
Tell us.
Do you have a recent research paper or an idea for a science/technology-themed article that you'd like to tell us about?
GET IN TOUCH