Good AI, bad AI
-
- from Shaastra :: vol 03 issue 10 :: Nov 2024
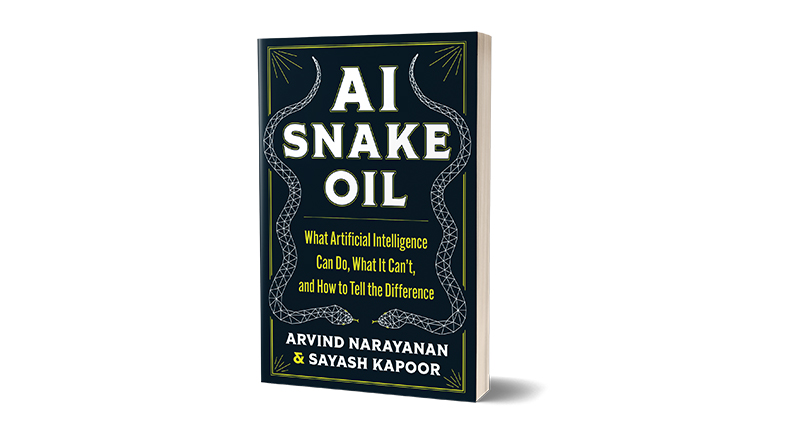
A useful primer on artificial intelligence, which flags the hype around it.
Despite the clickbait title, this is a serious, yet non-technical, review of artificial intelligence (AI), aimed at a lay readership. The authors run a newsletter, from where they derived the title. Arvind Narayanan and Sayash Kapoor are computer scientists at Princeton University, and net-positive in their attitude to AI. But as domain experts, they can also point out issues and misconceptions that are concerning.
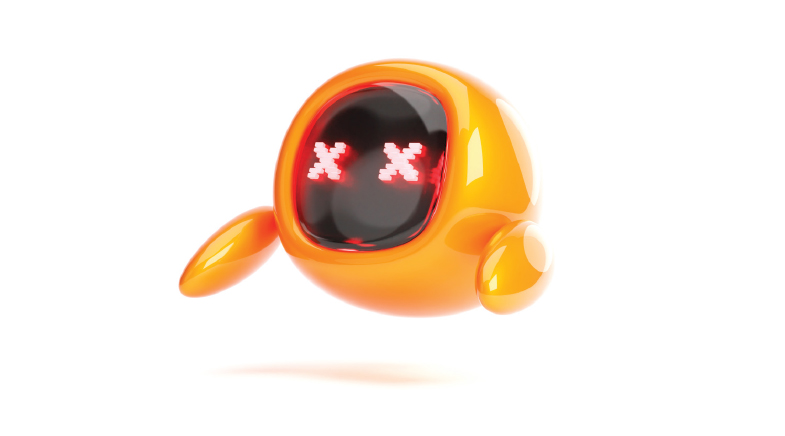
One way of summarising the themes of the book is that it flags hype. Any industry will hype new tools for obvious reasons, and media and social media usually amplify corporate hype. While mediapersons may do this in good faith, they may also do it in order to maintain relationships and access. The authors confess to being irritated by clickbait headlines and clichéd metaphors about "alive" AI, which is of course a little ironic.
One huge issue is lack of replicability in AI research. The book cites a study that claimed that none of a set of 400 studies on AI from leading peer-reviewed publications satisfied all the criteria (like sharing code and data) for reproducibility. Most papers satisfied merely 20-30% of reproducibility requirements, "making it hard to even investigate if results were reproducible", the authors note. This leads to a cascade of further concerns, given the increasing reliance on AI in various STEM disciplines.
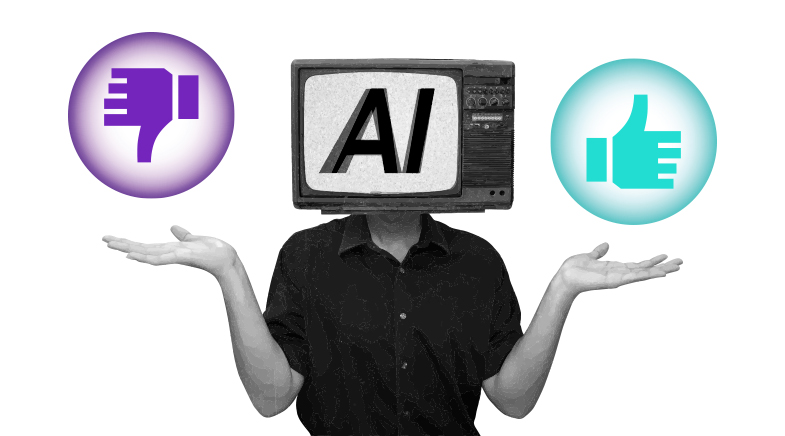
Another problem is data leakage (which also leads to issues of overfitting of models). If an algo is tested on the same or very similar data that it was trained on, it's like leaking the answers to a quiz. Models developed with high leakage may underperform traditional tools (like age-old regression analysis) with real-world data.
Since commercial entities won't share code and training data, how can you even evaluate algos? If you knew a tool was trained on data from Quora or Reddit, would you be comfortable asking it medical questions?
AI is also often seen as a miracle fix for broken domains like education, justice systems, and so on. AI comes with a branding of efficiency. But broken domains usually have many other inherent issues, in addition to lack of efficiency and, thus, performance doesn't live up to hype.
Moreover, AI is a label used for a broad range of things, and few laypersons know the differences between types of AI. This leads to misleading expectations about its utility. This book explains some of the differences between generative AI and predictive AI.
GENERATIVE VS PREDICTIVE
The authors are positive about generative AI, even while acknowledging that it can be a force multiplier for fakery and plagiarism. They seem to believe the potential impact of large language models (LLMs) is so vast that it is perhaps even understated by the current expectations!
But they are much less comfortable with predictive AI and cite horror stories. Predictive AI is a black box that can perpetrate existing biases. Applying predictive AI to decisions in hiring, or in the justice system, insurance and welfare, often hurts minorities and the poor, according to the authors. For example, an algorithm used in the Netherlands to predict potential welfare fraud wrongly targeted women, and immigrants who didn't speak Dutch.
The book suggests that predictive AI should have strict human oversight; indeed, legislation in the European Union tilts in this direction. Policymakers should pay heed to similar arguments about predictive AI.
Many of us are curious about AI, or afraid of it, or both. Yet, we see it proliferate at great speed in our personal lives and work spheres. Since you cannot avoid AI, you need to come to terms with the proliferation – and the book helps.
In general, the book helps readers develop a basic understanding of AI. That may spur the more curious into looking for details on machine learning, LLMs, neural networks, transformers, training data sources, and so on. More educated users should, in turn, be more selective and sceptical about AI deployment, and, thus, less likely to enter the hype bubble.
The authors' newsletter is also worth tracking. As the book inevitably becomes dated, the newsletter will keep readers updated. While the book has its flaws, it reminds us to be critical, while acknowledging the transformative impact of AI. Readers will become more aware of AI's limitations and be more likely to ask the right questions about its utility.
Devangshu Datta is a consulting editor and columnist with a focus on STEM and finance.
Have a
story idea?
Tell us.
Do you have a recent research paper or an idea for a science/technology-themed article that you'd like to tell us about?
GET IN TOUCH