The sound of bad judgments
-
- from Shaastra :: vol 01 edition 02 :: Jul - Aug 2021
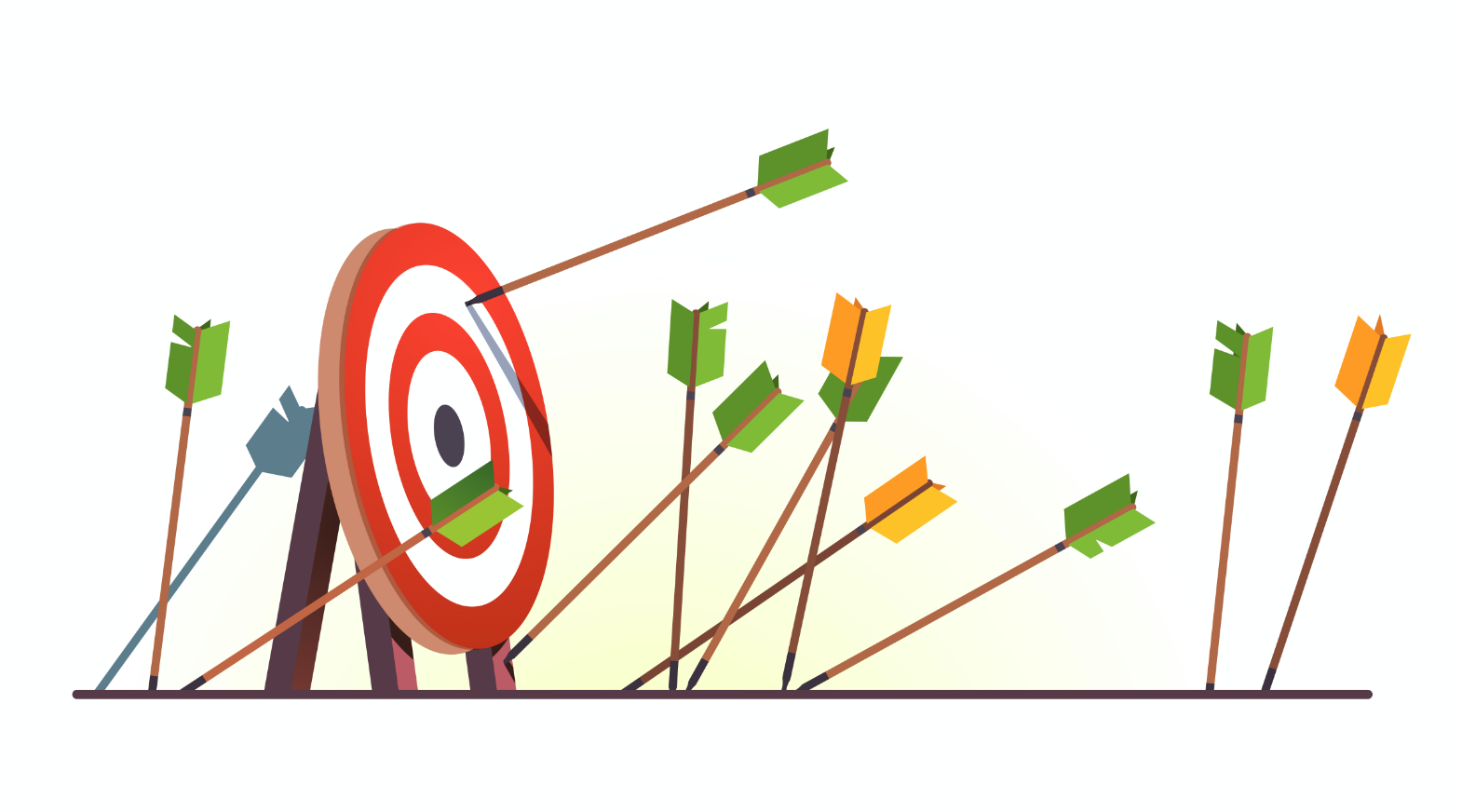
Strategies to shut out 'noise', or unwanted variability in judgments, which imposes high economic and social costs.
Two research papers published in a recent issue of Surgery journal starkly illustrate one of the more inexplicable idiosyncrasies of medical science: the contrasts in the judgments of two sets of professionals while looking at the same data. The professionals had been called upon to decide whether the use of a particular medical equipment during surgery to remove an inflamed appendix would increase or decrease the chance of an infection. They drew diametrically opposite conclusions even though their respective reasoning was rooted in the same data pool.
Diversity of opinions among medical professionals is, of course, highly consequential - since they deal with life-and-death matters. But such divergence is common enough in other fields as well. In the insurance industry, for instance, underwriters quote premiums for risks, and claims adjusters assess the value of future claims. While they may rely on actuarial science models, in the real world, their judgment calls, which hold enormous financial consequences for the insurance firm, have been known to vary in a wide range. And in the realm of criminal sentencing, there are countless instances of two people, convicted of the same crime, receiving vastly different penalties. On occasion, judicial outcomes hinge on frivolous considerations: whether it is a hot day, how the local football team fared the previous day, or even whether the hearing was held after a meal break!
In some life situations, of course, variability in professional judgments is not unwelcome - and may even be desirable. When multiple teams of researchers are working to develop a COVID-19 vaccine, say, we would want them to approach the problem from different angles. We also expect diversity of opinions from film critics or from wine-tasters, even when they've sampled the same produce. But the problem arises with unwanted variability in professional judgments, particularly among people who are expected to agree. Psychologist Daniel Kahneman, business strategist Olivier Sibony and legal scholar Cass R. Sunstein call this noise.
BIAS vs NOISE
As the authors frame it, noise is different from bias, even though both are different components of error. Bias is a systematic deviation; if the errors in judgements all follow in the same direction - for instance, if ethnic minorities are routinely penalised in disproportionate numbers - that reflects bias: it manifests itself in an archer who consistently misses the bull's-eye, but all of whose arrows are clustered in one off-centre area. Noise, on the other hand, is variability of error, where the errors in judgements follow in many directions. It is random scatter, as happens with a loose cannon: in the pictorial illustration above, it's when an archer peppers the target without a pattern, to the point where it's impossible to say where the next arrow might land.
Organisations and professional groups may be afflicted by both bias and noise, but whereas they may even be aware of their inherent bias, they don't really know the extent to which they are susceptible to noise.
Showcasing many real-world examples of noise and its effect at work and in broader society, Kahneman, Sibony and Sunstein detail many tools to cancel it out, improve judgements and prevent error by employing so-called decision hygiene techniques. Two of these noise-reduction strategies merit particular attention, given that the authors place enormous faith in them. The first is by aggregating multiple independent judgments. That is, by drawing on the "wisdom of crowds" - or crowdsourcing opinions and then averaging out different judgments. But that can work only if the crowd constituents are immune to 'groupthink' pressure, which is a challenge as exemplified by conformity experiments.
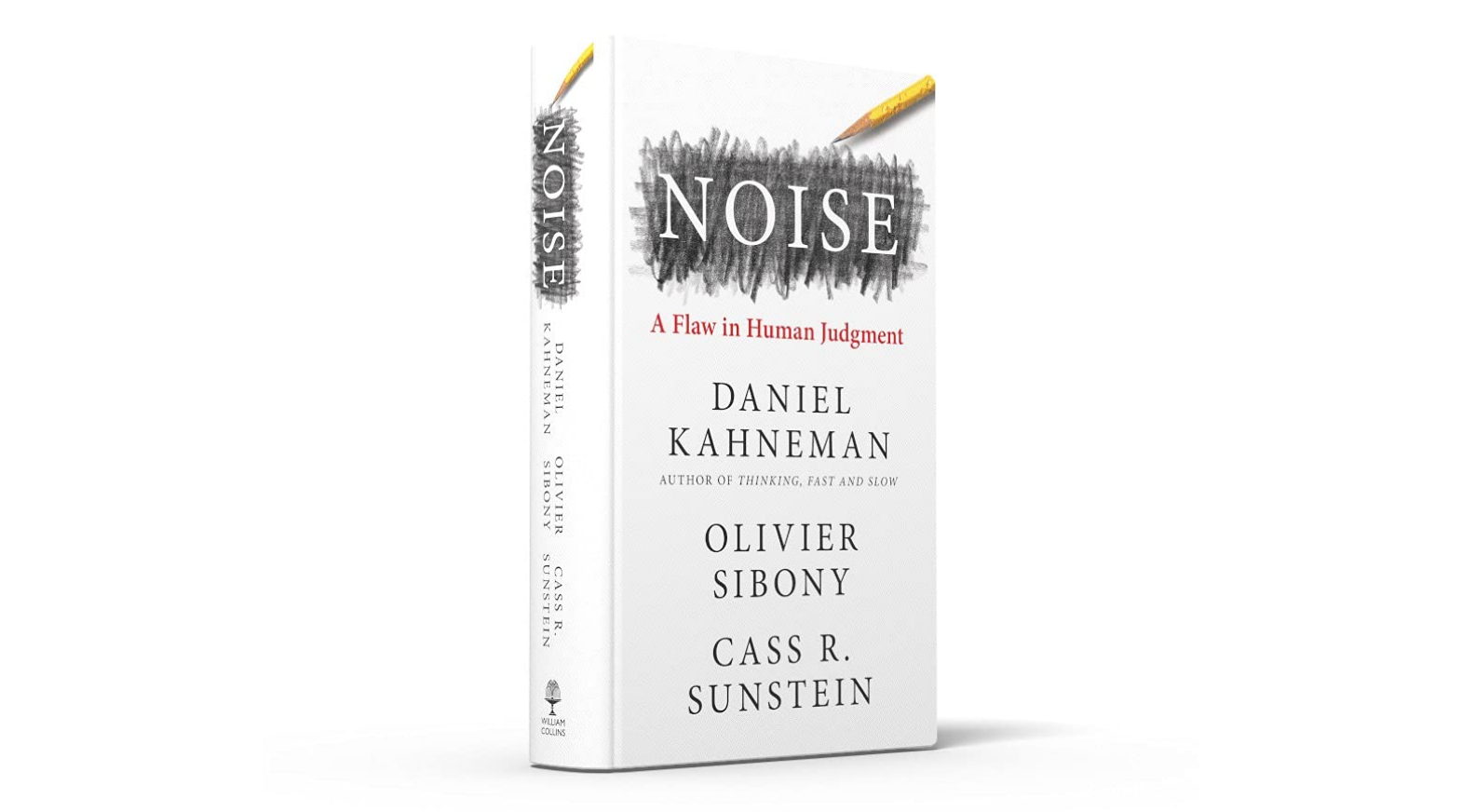
The other strategy - of harnessing rules, formulas and algorithms over humans while making predictions - is a recurring theme for the authors, although they fleetingly acknowledge the malefic effect of "algorithm bias". To give them their due, the formula-based noise-cancellation strategy did demonstrably work for a while, in specific domains. For instance, one of the broadest sets of prison sentencing reforms in the U.S., propelled in the 1970s by judge Marvin Frankel, saw the dilution of much of the "unfettered discretion" that judges and parole authorities enjoyed while imposing and implementing sentences. In its place, the reforms introduced mandatory "sentencing guidelines" - a check-list of sorts - that established a restricted range for criminal sentences.
But the seeds of failure were embedded in those very provisions. Judges bristled at the dilution of their discretionary powers, and pushed back against their implementation. Over time, the guidelines were made 'advisory', which effectively rendered them toothless.
SILENCE IS IMPRACTICAL
In other words, as Kahneman, Sibony and Sunstein point out, the right level of noise in an organisation or society may not be zero. In some realms, it is infeasible - or too expensive - to eliminate noise. Or, as the experience of shackling judges’ discretionary powers established, efforts to eliminate noise may create the perception that humans were being reduced to automatons, and dampen morale among critical stakeholders.
Even so, the authors make a persuasive case for organisations, and the pillars of civil society, to embrace these noise-reduction strategies - in the interest of fairness and efficiency. Their prose may occasionally border on the dense, but in training their collective attention on a little-researched aspect of flawed decision-making, they light up the path away from the shadows of the mind where cognitive biases - and noise - play tricks.
Have a
story idea?
Tell us.
Do you have a recent research paper or an idea for a science/technology-themed article that you'd like to tell us about?
GET IN TOUCH