The chemistry chatbot
-
- from Shaastra :: vol 03 issue 02 :: Mar 2024
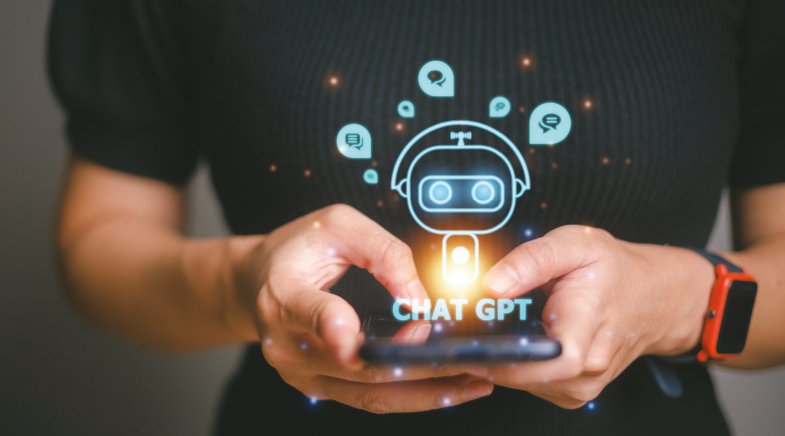
A refined GPT-3 model takes on chemistry tasks, rivalling conventional machine learning — even with smaller datasets.
An article on GPT-3 being able to do a math problem that it could not have known shook up Berend Smit, Professor at Swiss Federal Institute of Technology Lausanne (EPFL), and his then-PhD student Kevin Jablonka. GPT-3 (Generative Pre-trained Transformer 3), a powerful natural language processing model developed by OpenAI, is capable of generating human-like text based on input prompts. Jablonka, now at the Friedrich Schiller University Jena in Germany, wondered if GPT-3's success in mathematics could be replicated for chemistry.
GPT-3's knowledge of specific areas of chemistry was limited to what was available online. Sparked by their initial motivation, the researchers trained and tested GPT-3 for predictive chemistry. One of the areas Jablonka and Smit focused on was high entropy alloys (HEA) – alloys of two or more metals with significant applications as structural materials, biomaterials or even parts of a nuclear reactor. They trained GPT-3 with a simple string of known examples, phrased in a question–answer format.
"Of course, the model is not perfect, so we use a test set of results not seen by the model to estimate the accuracy," explains Smit. In a recent Nature Machine Intelligence paper (bit.ly/LLMChemistry), the researchers reported that GPT-3 trained on 50 data points performed at par with predictive chemistry models trained on more than 1,000 data points. They proceeded to test the limits of their refined GPT-3 model beyond HEA in other areas like solubility in water or oils, performance of organic photovoltaics, heat capacities and chemical reaction yield. They found that their model performed equally well or better than traditional chemistry machine learning models trained on smaller datasets. These models took over GPT-3 only when trained on large amounts of data.
"It sets a benchmark for future machine learning studies: one should at least be better than GPT-3."
A. Anoop, Professor of Digital Sciences at the Digital University Kerala in Thiruvananthapuram, who was not involved in the study, says, "The application of machine learning in science is growing. The latest trend is to use the large language models in chemistry." Anoop, who had co-organised a national meeting on machine learning for molecular sciences recently, adds that, "This is a prototype of many such developments to come."
Smit sees the model's simplicity, text-based interaction and ease of use as a breakthrough. "It sets a benchmark for future machine learning studies: one should at least be better than GPT-3," says Smit, adding that any chemist can use this to explore or optimise things, and "it may not be very accurate if the data set is too small, but it is our experience it is always better than a random guess."
Have a
story idea?
Tell us.
Do you have a recent research paper or an idea for a science/technology-themed article that you'd like to tell us about?
GET IN TOUCH