PINNing hopes on the future
-
- from Shaastra :: vol 04 issue 03 :: Apr 2025
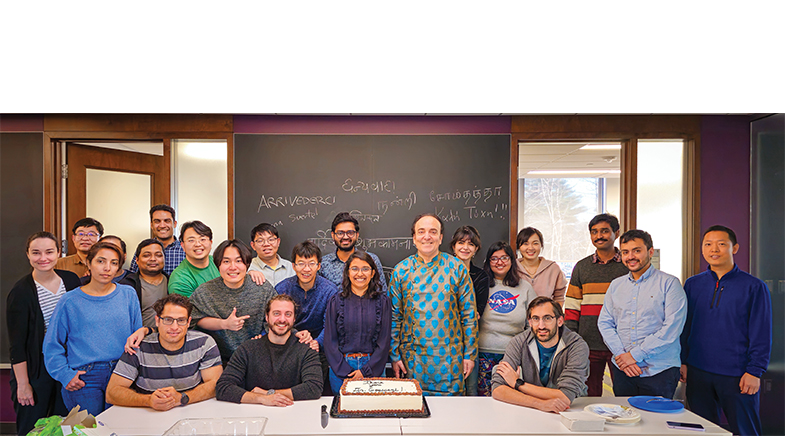
Physics-informed neural networks are emerging as the future of medical science — enabling cancer detection and drug discovery.
George Karniadakis calls it the "Ferrari of the sea". About a decade ago, the Professor of Applied Mathematics at Brown University, U.S., started working on a project to create a high-speed sea vehicle for the American defence sector. The craft would glide and skim the water's surface, supported by sharp hydrofoils. To achieve this, his team developed mathematical models and algorithms to analyse the complex three-dimensional environment surrounding the vessel. One of the biggest challenges involved solving complex partial differential equations that described fluid flow in computational fluid dynamics. When standard machine learning methods failed to deliver the desired outcome, the team incorporated the physics of fluid dynamics into their training data. This approach led them to develop physics-informed neural networks (PINNs) (bit.ly/PINN-first), which helped them achieve their goal of building the superfast craft.
The method, which had once evoked scepticism, is proving impactful in health and biomedical sciences. From enabling the effective detection and diagnosis of cancer to drug discovery and from helping devise medical devices to imaging, it is emerging as the future of medical science.
In 2022, Nazanin Ahmadi Daryakenari, a biomedical researcher from Iran, joined Karniadakis as a PhD scholar and started exploring the effectiveness of PINN in biomedical sciences. "There's not a single field — from agriculture to astrophysics — where PINN has not been used," Karniadakis says. "Nazanin may have been the first to apply it in quantitative pharmacology."
Simplified models, Daryakenari says, can sometimes fail to explain complex systems. For instance, a laboratory (in vitro) study might follow a simpler model, but when applied to real biological systems (in vivo), the actual behaviour may be far more complex than the model predicts. This difference occurs because the simplified equation might miss important factors. Most models, for example, usually measure glucose levels in the blood and insulin. However, they often ignore interstitial insulin — found in the fluid around cells — because it is difficult to measure such levels directly from the body. But interstitial insulin plays a crucial role in the way a system behaves.
To address these gaps, the Brown University group in 2023 developed AI-Aristotle, a PINN system that incorporates physics equations of a fluid's dynamics (bit.ly/Aristotle-dl). The group's study captures the dynamical and stubborn behaviour of tumour growth. The group observed that after the first dose of a required medicine, sensitive tumour cells were rapidly destroyed. With subsequent doses, however, fewer sensitive cells remained, while resistant cells grew, reducing the drug's impact. This phenomenon, known as pharmacokinetic tolerance, occurs as the body adapts to eliminate and distribute a drug more efficiently. While traditional models rely on fixed parameters, Daryakenari's team used a two-compartment model with time-varying parameters to better track the real-time evolution of the tumour cells.
Karniadakis explains that the team improved traditional models in two main ways. First, instead of using fixed values for interactions, it used functions that could change over time. Second, it allowed these values to vary with time, enabling the model to capture gradual changes more effectively. It also used fractional calculus to account for delays in the system's response. Combining these ideas with neural networks identified missing parts in the equations. PINN helped the scientists find unknowns, doing away with the need for expensive and complex experiments and developing a new model of drug absorption and memory effects (bit.ly/Two-compartment).
UNMASKING HIDDEN SITES
Mixing artificial intelligence (AI) with physics leads to such conformational states, which can steer specific therapeutics, points out Soumendranath Bhakat, Scientist at Washington University in St. Louis and Co-Founder of the AlloTec Bio company, which explores protein mechanisms for therapeutics. Bhakat's research domain is drug discovery using generative AI methods, such as AlphaFold, in protein structure prediction. He has found that AlphaFold and similar tools can help predict a single static structure. Bhakat and his team incorporated physical laws, such as Newton's laws of motion, into AlphaFold (bit.ly/Cryptic-pocket), which enabled them to create a 360°-view of proteins.
This exposed the proteins' hidden structures, such as cryptic pockets. Cryptic pockets, he says, are hidden sites that exist in proteins and are linked to diseases and conditions such as cancer, antibiotic resistance, and malaria. Targeting these pockets could improve drug selectivity, reducing side effects. He points out that most human proteins are not naturally "druggable" — only 10-20% are. Identifying cryptic pockets may drastically expand the number of "druggable" targets. By running molecular simulations on these varied structures, they could explore a broader range of protein movements, increasing the chances of discovering cryptic pockets.
Physics plays a crucial role in this research. While AI models such as AlphaFold predict static structures, detecting these rare cryptic pockets requires understanding the dynamic movements of proteins. By incorporating Newton's laws of motion and stochastic differential equations in molecular simulations, they could better capture these rare structural shifts.
He notes that while AI models excel at finding patterns in data, they lack an intrinsic understanding of physical laws. Therefore, combining AI predictions with physics-based simulations allows the system to refine its understanding and improve accuracy.
Bhakat mentions that his team is collaborating with pharmaceutical companies and academic institutions to identify cryptic pockets for novel drug development. He believes this will accelerate breakthroughs in therapeutic discovery in the coming years.
CANCER DETECTION DEVICE
Mechanobiologist Suman Chakraborty, Professor at the Indian Institute of Technology (IIT) Kharagpur, believes this combination of physical laws and patient data, particularly in healthcare, can be enhanced by incorporating clinically validated inputs for feature extraction and classification. In 2022, Chakraborty and his team developed a low-cost, hand-held oral cancer detector. They used PINN to analyse thermal imaging data to detect this form of cancer. The process involves capturing thermal images using a camera and interpreting blood perfusion data based on heat transfer principles and physics-based equations. However, he notes that while blood perfusion data are linked to vascularity, the interpretation remains challenging because similar perfusion patterns can arise due to multiple factors. To deal with this, additional clinical inferences from doctors are incorporated for feature extraction from the thermal images. These clinical inputs help refine the interpretation and serve as feedback for training the algorithm. Over time, as more images with corresponding auxiliary inputs are added, the algorithm improves its ability to classify cases as precancerous, cancerous, or otherwise.
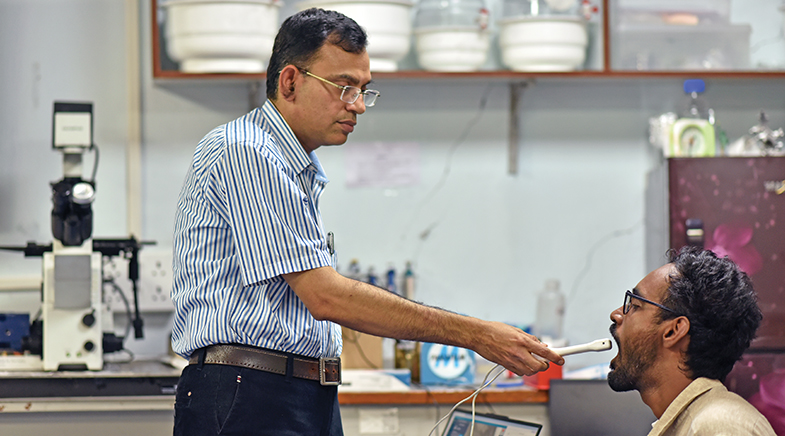
Chakraborty emphasises that besides fundamental physics principles, incorporating patient-specific details — such as symptoms, medical history, and lifestyle issues such as tobacco use — along with boundary conditions, including mouth temperature and humidity, can help doctors make more informed interpretations. This approach, he adds, is particularly beneficial in scenarios where physics alone cannot provide definitive answers, often due to data limitations, uncertainties, or the complexity of biological systems. By incorporating machine learning (ML), uncertainties in interpretation — arising from physiological variations — can be addressed. Chakraborty says that this combination of physics and data science is gaining popularity as a more robust and practical approach.
Physics-informed medical image analysis is an advanced computational method.
He also points out that scientific communities often have two extreme viewpoints: one that believes AI alone can solve all problems and another that holds physics-based theoretical models as sufficient. However, he argues, a balanced integration of the two perspectives offers the most practical solutions.
MEDICAL IMAGING
Physics-informed medical image analysis (PIMIA) is an advanced computational method integrating physics principles with medical image processing tasks. "In a scenario when you have no data, you have to rely completely on physics," says Chayan Banerjee, a postdoctoral research fellow at Queensland University of Technology, Australia. In a 2024 survey paper, based on 70 research papers, Banerjee and his collaborators looked at the potential of PINN in future medical imaging. The researchers show that physics equations and mathematical models can help them understand processes in the human body and medical imaging. While these may not capture every detail, they still provide useful insights for analysing medical images, Banerjee says.
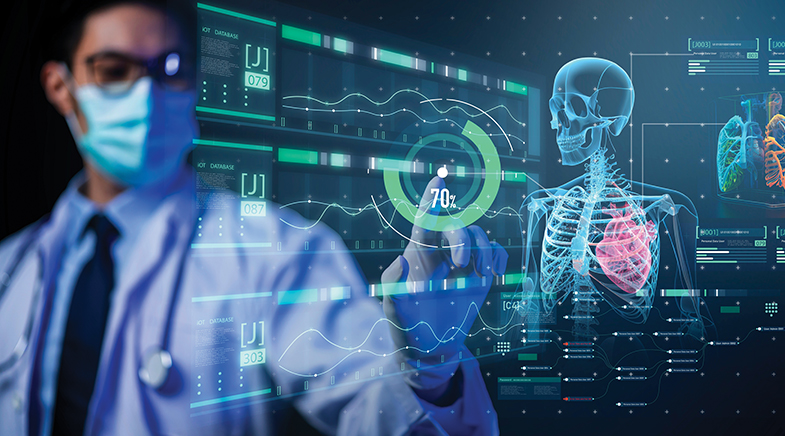
Unlike purely data-driven approaches, PIMIA combines domain knowledge about the physical processes behind image formation with ML algorithms. This helps improve the accuracy, robustness, and reliability of medical imaging applications such as MRIs, CT scans, X-rays, and ultrasound.
The survey paper highlights recent studies that have integrated physics-based information into medical image analysis to enhance diagnostic accuracy and image quality. Researchers have developed physics-informed deep learning for Dual-Energy Computed Tomography (DECT). They have devised a convolutional neural network (CNN) that combines the physics of DECT imaging with anatomical context to improve material differentiation in brain scans. Their method addressed the limitations of traditional physics-based approaches, which often produce images with low signal-to-noise ratios. By integrating deep learning with physics principles, their CNN generated clearer images that improved the distinction between materials such as calcium and iodine (bit.ly/DET-paper).
Digital human twins help predict disease risks, improve diagnoses, guide treatment plans by integrating patient data, and create realistic organ models for surgical planning.
In another study, researchers introduced physics-informed motion correction in Gradient Echo (GRE), which leads to improved MRI scans, especially those affected by patient movement. It identifies and removes the blurry or distorted data caused by motion and helps create sharper images. This method also accelerates the scanning process by reducing the need for extra data collection, cutting scan times by over 40%.
Banerjee also refers to the emergence of digital human twins (DHTs), which help predict disease risks, improve diagnoses, and guide treatment plans by integrating diverse patient data. DHTs create realistic organ models for precise surgical planning and simulate patient responses to targeted therapies. They also support drug development by predicting drug effects, making the process safer and more efficient. Such tools available in the market include Siemens Digital Twin for Healthcare, Ansys medini analyze and NVIDIA Omniverse. NVIDIA Omniverse traces real-time data from a CT scan or an MRI and produces 3D visuals for surgical planning, collaboration, and simulations. The technology leads to detailed 3D images from a CT scan.
These developments highlight how combining physics-based models with deep learning can significantly improve medical imaging methods, leading to better diagnostics and medical procedures.
FUTURE OF MEDICINE
"In molecular development, physics-based models strive to recreate the complete environment — including proteins, ions, and solvents — as each element significantly impacts the system's behaviour, similar to how a crash simulation models a car's entire structure and environment," says Jayant K. Singh, Professor, Department of Chemical Engineering at IIT Kanpur. Just before the COVID-19 pandemic, Singh initiated a start-up, Prescience Insilico, in Bengaluru, which focused on drug design when the pandemic spread. Its first task was repurposing existing drugs to suppress the virus's impact. Within six months, it developed tools and methodologies, successfully identifying four potential drugs, three of which later entered clinical trials.
Singh explains that his team used physics-informed machine learning (PIML) throughout the process. This approach integrated physics-based information, which had been a core part of their work. Singh's start-up is developing sustainable formulations for fast-moving consumer goods by reducing the water content in the products and replacing petroleum-based molecules with greener alternatives. Using physics-based models and digital twins for hair, skin, and dental surfaces, they simulate product behaviour to reduce experiment costs by 50%. Their technology also predicts formulation stability, ensuring long-term product quality.
The start-up's software platform — called PRinS3 — has been designed to streamline research and accelerate drug discovery. Singh holds that as India lacked such software tools, researchers developed indigenous solutions rather than relying on foreign software. The company aims to develop digital twins for skin, dental, and hair systems — virtual models replicating their real-world counterparts. By combining genetic, physiological, and environmental data with advanced computational models and ML, these simulations offer personalised insights for predicting outcomes, planning treatments, and optimising care strategies.
Healthcare relies on experience and evidence because the human body is too complex for a one-size-fits-all approach. Personalised medicine is becoming more and more important, requiring patient-specific data and basic physical principles from physiology or medical imaging. "The combination of patient data, some basic physics rules, and the wisdom of the doctors — I think that is where the future of healthcare is getting into," Chakraborty says.
See also:
Have a
story idea?
Tell us.
Do you have a recent research paper or an idea for a science/technology-themed article that you'd like to tell us about?
GET IN TOUCH